Discover
Practical AI: Machine Learning, Data Science, LLM
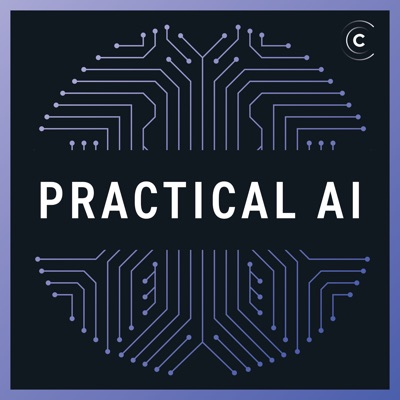
Practical AI: Machine Learning, Data Science, LLM
Author: Changelog Media
Subscribed: 5,762Played: 158,081Subscribe
Share
© All rights reserved
Description
Making artificial intelligence practical, productive & accessible to everyone. Practical AI is a show in which technology professionals, business people, students, enthusiasts, and expert guests engage in lively discussions about Artificial Intelligence and related topics (Machine Learning, Deep Learning, Neural Networks, GANs, MLOps, AIOps, LLMs & more).
The focus is on productive implementations and real-world scenarios that are accessible to everyone. If you want to keep up with the latest advances in AI, while keeping one foot in the real world, then this is the show for you!
The focus is on productive implementations and real-world scenarios that are accessible to everyone. If you want to keep up with the latest advances in AI, while keeping one foot in the real world, then this is the show for you!
296 Episodes
Reverse
This week, Chris is joined by Gregory Richardson, Vice President and Global Advisory CISO at BlackBerry, and Ismael Valenzuela, Vice President of Threat Research & Intelligence at BlackBerry. They address how AI is changing the threat landscape, why human defenders remain a key part of our cyber defenses, and the explain the AI standoff between cyber threat actors and cyber defenders.
Elham Tabassi, the Chief AI Advisor at the U.S. National Institute of Standards & Technology (NIST), joins Chris for an enlightening discussion about the path towards trustworthy AI. Together they explore NIST's 'AI Risk Management Framework' (AI RMF) within the context of the White House's 'Executive Order on the Safe, Secure, and Trustworthy Development and Use of Artificial Intelligence'.
We are on the other side of "big data" hype, but what is the future of analytics and how does AI fit in? Till and Adithya from MotherDuck join us to discuss why DuckDB is taking the analytics and AI world by storm. We dive into what makes DuckDB, a free, in-process SQL OLAP database management system, unique including its ability to execute lighting fast analytics queries against a variety of data sources, even on your laptop! Along the way we dig into the intersections with AI, such as text-to-sql, vector search, and AI-driven SQL query correction.
Workflow orchestration has always been a pain for data scientists, but this is exacerbated in these AI hype days by agentic workflows executing arbitrary (not pre-defined) workflows with a variety of failure modes. Adam from Prefect joins us to talk through their open source Python library for orchestration and visibility into python-based pipelines. Along the way, he introduces us to things like Marvin, their AI engineering framework, and ControlFlow, their agent workflow system.
As Argilla puts it: "Data quality is what makes or breaks AI." However, what exactly does this mean and how can AI team probably collaborate with domain experts towards improved data quality? David Berenstein & Ben Burtenshaw, who are building Argilla & Distilabel at Hugging Face, join us to dig into these topics along with synthetic data generation & AI-generated labeling / feedback.
We are constantly hearing about disillusionment as it relates to AI. Some of that is probably valid, but Mike Lewis, an AI architect from Cincinnati, has proven that he can consistently get LLM and GenAI apps to the point of real enterprise value (even with the Big Cos of the world). In this episode, Mike joins us to share some stories from the AI trenches & highlight what it takes (practically) to show what is possible, doable & scalable with AI.
Seems like we are hearing a lot about GraphRAG these days, but there are lots of questions: what is it, is it hype, what is practical? One of our all time favorite podcast friends, Prashanth Rao, joins us to dig into this topic beyond the hype. Prashanth gives us a bit of background and practical use cases for GraphRAG and graph data.
Recently the company stewarding the open source library scikit-learn announced their seed funding. Also, OpenAI released "o1" with new behavior in which it pauses to "think" about complex tasks. Chris and Daniel take some time to do their own thinking about o1 and the contrast to the scikit-learn ecosystem, which has the goal to promote "data science that you own."
Dinis Cruz drops by to chat about cybersecurity for generative AI and large language models. In addition to discussing The Cyber Boardroom, Dinis also delves into cybersecurity efforts at OWASP and that organization's Top 10 for LLMs and Generative AI Apps.
GenAI is often what people think of when someone mentions AI. However, AI is much more. In this episode, Daniel breaks down a history of developments in data science, machine learning, AI, and GenAI in this episode to give listeners a better mental model. Don't miss this one if you are wanting to understand the AI ecosystem holistically and how models, embeddings, data, prompts, etc. all fit together.
How do you systematically measure, optimize, and improve the performance of LLM applications (like those powered by RAG or tool use)? Ragas is an open source effort that has been trying to answer this question comprehensively, and they are promoting a "Metrics Driven Development" approach. Shahul from Ragas joins us to discuss Ragas in this episode, and we dig into specific metrics, the difference between benchmarking models and evaluating LLM apps, generating synthetic test data and more.
If you have questions at the intersection of Cybersecurity and AI, you need to know Donato at WithSecure! Donato has been threat modeling AI applications and seriously applying those models in his day-to-day work. He joins us in this episode to discuss his LLM application security canvas, prompt injections, alignment, and more.
You might have heard that "AI is only as good as the data." What does that mean and what data are we talking about? Chris and Daniel dig into that topic in the episode exploring the categories of data that you might encounter working in AI (for training, testing, fine-tuning, benchmarks, etc.). They also discuss the latest developments in AI regulation with the EU's AI Act coming into force.
There is an increasing desire for and effort towards GPU alternatives for AI workloads and an ability to run GenAI models on CPUs. Ben and Greg from Intel join us in this episode to help us understand Intel's strategy as it related to AI along with related projects, hardware, and developer communities. We dig into Intel's Gaudi processors, open source collaborations with Hugging Face, and AI on CPU/Xeon processors.
We discussed "🥦 Broccoli AI" a couple weeks ago, which is the kind of AI that is actually good/healthy for a real world business. Bengsoon Chuah, a data scientist working in the energy sector, joins us to discuss developing and deploying NLP pipelines in that environment. We talk about good/healthy ways of introducing AI in a company that uses on-prem infrastructure, has few data science professionals, and operates in high risk environments.
This week Daniel & Chris hang with repeat guest and good friend Demetrios Brinkmann of the MLOps Community. Together they review, debate, and poke fun at the 2024 Gartner Hype Cycle chart for Artificial Intelligence. You are invited to join them in this light-hearted fun conversation about the state of hype in artificial intelligence.
In the midst of the demos & discussion about OpenAI's GPT-4o voice assistant, Kyutai swooped in to release the *first* real-time AI voice assistant model and a pretty slick demo (Moshi). Chris & Daniel discuss what this more open approach to a voice assistant might catalyze. They also discuss recent changes to Gartner's ranking of GenAI on their hype cycle.
Daniel & Chris explore the advantages of vector databases with Roie Schwaber-Cohen of Pinecone. Roie starts with a very lucid explanation of why you need a vector database in your machine learning pipeline, and then goes on to discuss Pinecone's vector database, designed to facilitate efficient storage, retrieval, and management of vector data.
We've had representatives from Stanford's Institute for Human-Centered Artificial Intelligence (HAI) on the show in the past, but we were super excited to talk through their 2024 AI Index Report after such a crazy year in AI! Nestor from HAI joins us in this episode to talk about some of the main takeaways including how AI makes workers more productive, the US is increasing regulations sharply, and industry continues to dominate frontier AI research.
Daniel & Chris engage in an impromptu discussion of the state of AI in the enterprise. Then they dive into the recent _Apple Intelligence_ announcement to explore its implications. Finally, Daniel leads a deep dive into a new topic - Advanced RAG - covering everything you need to know to be practical & productive.
Top Podcasts
The Best New Comedy Podcast Right Now – June 2024The Best News Podcast Right Now – June 2024The Best New Business Podcast Right Now – June 2024The Best New Sports Podcast Right Now – June 2024The Best New True Crime Podcast Right Now – June 2024The Best New Joe Rogan Experience Podcast Right Now – June 20The Best New Dan Bongino Show Podcast Right Now – June 20The Best New Mark Levin Podcast – June 2024
PendDown: 100-200
The concept of the Practical AI show sounds really interesting! It's so important to understand discussions around AI and related topics and apply them in real-world scenarios. If you're also interested in financial services, make sure to check out this link: https://myconveyancingmatters.co.uk/services/mortgage-product-transfer/ which provides helpful information on mortgage product transfers.
thanks for the episode, It really gave a good understanding of the different areas. sometimes it is good to dial back the technical to summarise the basics.
Please consider removing the annoying background music while guests are speaking (27.30) so we can listen to the content and not 60 seconds of sponsored ad intro music
The file is damaged and won't play properly.
🔴💚Really Amazing ️You Can Try This💚WATCH💚ᗪOᗯᑎᒪOᗩᗪ👉https://co.fastmovies.org
✅ CLICK HERE Full HD 1080p 4K👉👉https://co.fastmovies.org
Thank for podcast and it's was very useful for me
great talks. I loved the concluding part.
Processing data is a pretty complex process. This podcast did a pretty good job explaining it. But if you want to learn something more about labeling and annotation, look here https://marketbusinesstimes.com/data-labeling/. In this article, you can find some decent information about raw data processing in machine learning which can really help you down the road.
I got a call yesterday from a friend of mine. He told me that he has to do courses in Machine Learning, Data Science. After that I shared the link of this post with him. Because Machine Learning, Data Science and chat gpt https://supercharged-by-ai.com/ service were told on this post. A website link was also given here. You can get the initial information of AI from that website.
I noticed that the educational niche market is now rapidly developing due to companies such as https://geniusee.com/edtech that have simplified the creation and development of software or web products for training. Now there are already good strategies for creating such things.
An alternative to DigitalOcean hosting: https://scalegrid.io/mysql/digitalocean.html
Great introduction to what's going on in AI. Already started on getting MachineBox up and running. Looking forward to my commutes so I can learn some more! Mark Cund (@AluminumBlonde)