Exploring AI Modeling: Key Concepts and Applications
Digest
Andrew Madsen, a tech evangelist at Dremio, shares his journey into the world of data analytics. He explains how his experience in finance, where he had to make data-driven decisions, led him to pursue a career in data analysis. He highlights the challenges he faced transitioning from a high-paying finance role to a data analytics position, including the lack of experience and the need to work two full-time jobs to build his data resume. Madsen emphasizes the importance of staying technical even in leadership roles and shares his approach to staying up-to-date with the latest technologies. He discusses the differences between teaching data analytics in academia and working in the industry, noting that while academia focuses on fundamentals, companies often require more advanced skills. He expresses his excitement about the potential of data lake houses for real-time AI and analytics, explaining the advantages over traditional data warehouses. Madsen also shares his insights on the future of AI, predicting a shift towards niche AI models that excel in specific tasks rather than large, general-purpose models. He discusses the importance of data quality for AI, highlighting the need for consistent definitions and accurate data. He also touches on the potential of synthetic data for training AI models, expressing his reservations about its reliability. Madsen believes that open-source AI models have the potential to surpass proprietary models in the future, drawing parallels to the shift from SAS to Python and R in machine learning. He concludes by offering advice to those entering the field, encouraging them to start small and gradually build their skills. He also emphasizes the importance of staying up-to-date with the latest advancements in AI and data analytics.
Outlines
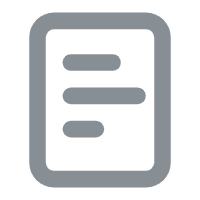
Introduction and Andrew's Journey into Data Analytics
This Chapter introduces Andrew Madsen, a tech evangelist at Dremio, and explores his journey into the field of data analytics. Andrew shares his background in finance and how his experience with data-driven decision-making led him to pursue a career in data analysis. He discusses the challenges he faced transitioning from finance to data analytics, including the lack of experience and the need to work two full-time jobs to build his data resume. He also emphasizes the importance of staying technical even in leadership roles and shares his approach to staying up-to-date with the latest technologies.
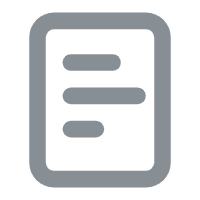
Data Lake Houses and the Future of AI
This Chapter delves into the concept of data lake houses and their potential for real-time AI and analytics. Andrew explains the advantages of data lake houses over traditional data warehouses, highlighting the ability to perform analytics and AI directly on raw data, reducing costs and improving efficiency. He discusses the role of Apache Iceberg and other columnar table formats in enabling this approach and the impact on the speed of AI development. He also shares his insights on the future of AI, predicting a shift towards niche AI models that excel in specific tasks rather than large, general-purpose models.
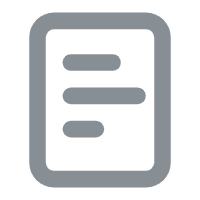
The Importance of Tech Evangelism
This Chapter focuses on the role of tech evangelists in bridging the gap between technical knowledge and real-world application. Andrew explains how tech evangelism differs from developer relations and marketing, emphasizing its focus on deeply understanding a product at a technical level and working with developers to effectively implement it. He discusses the growing importance of tech evangelism in the tech industry and how companies are increasingly seeking individuals with technical expertise and strong communication skills to build communities around their products.
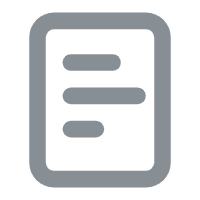
AI's Impact on Data Quality and Education
This Chapter explores the potential of AI to address challenges in data quality and education. Andrew discusses how AI can be used to categorize data, build semantic layers, and identify outliers, improving data quality and enabling better communication between technical and business teams. He also highlights the potential of AI to transform education by automating administrative tasks, enhancing lesson delivery, and empowering students to focus on higher-level learning.
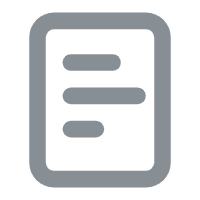
Common Misconceptions about AI and the Importance of Data Fundamentals
This Chapter addresses common misconceptions about AI and emphasizes the importance of data fundamentals. Andrew clarifies that AI is not a magic bullet and that its effectiveness depends heavily on the quality of the underlying data. He warns against relying on AI to solve problems without addressing fundamental data issues, such as inconsistent definitions and poor data quality. He stresses the need for strong data foundations to ensure that AI can be used effectively and avoid exacerbating existing problems.
Keywords
Tech Evangelism
Tech evangelism is a role in the tech industry that focuses on promoting and educating developers and other technical users about a specific product or technology. Tech evangelists are typically experts in the product or technology and have strong communication skills. They work to build communities around the product, provide technical support, and help developers implement the technology effectively. Tech evangelism is distinct from developer relations, which focuses on documentation and support, and marketing, which focuses on broader product promotion.
Data Lake House
A data lake house is a data architecture that combines the benefits of data lakes and data warehouses. It allows for the storage of both structured and unstructured data in a single location, while providing the ability to perform analytics and AI directly on the raw data. This approach eliminates the need to move data to a separate data warehouse, reducing costs and improving efficiency. Data lake houses are becoming increasingly popular as companies seek to leverage the power of AI and analytics on their data without the limitations of traditional data warehouses.
Apache Iceberg
Apache Iceberg is an open-source table format that enables efficient data access and management in data lakes. It provides a structured way to organize and query data stored in data lakes, making it possible to perform analytics and AI directly on the raw data. Iceberg's ability to omit unnecessary data during queries makes it particularly well-suited for large-scale data analysis and AI applications.
Generative AI
Generative AI is a type of artificial intelligence that focuses on creating new content, such as text, images, audio, and video. It uses machine learning algorithms to learn patterns from existing data and generate new data that resembles the original data. Generative AI has gained significant attention in recent years with the development of models like ChatGPT and DALL-E, which can generate human-quality text and images.
AGI (Artificial General Intelligence)
AGI, or Artificial General Intelligence, refers to a hypothetical type of AI that possesses human-level intelligence and can perform any intellectual task that a human can. It is a long-term goal of AI research, and there is ongoing debate about its feasibility and potential impact on society. While current AI systems are specialized in specific tasks, AGI aims to create AI systems that are capable of general problem-solving and learning.
Synthetic Data
Synthetic data is artificial data that is generated to mimic real-world data. It is often used in situations where real data is unavailable, expensive to collect, or contains sensitive information. Synthetic data can be used to train machine learning models, test software, and conduct simulations. However, there are concerns about the reliability of synthetic data and the potential for errors to propagate during training.
Hugging Face
Hugging Face is a company and community that focuses on open-source AI and machine learning. It provides a platform for sharing and collaborating on AI models, datasets, and code. Hugging Face is known for its large collection of pre-trained models, including GPT models, which can be used for various tasks such as text generation, translation, and question answering. Hugging Face's open-source approach has made it a popular resource for developers and researchers working in the field of AI.
OpenAI
OpenAI is a research and deployment company that focuses on developing and promoting friendly AI. It is known for its development of large language models, such as GPT-3 and ChatGPT, which have revolutionized the field of natural language processing. OpenAI has also made significant contributions to other areas of AI research, including robotics and reinforcement learning. The company's mission is to ensure that AI benefits all of humanity.
NVIDIA
NVIDIA is a technology company that specializes in graphics processing units (GPUs). Its GPUs are widely used in the field of AI and machine learning, as they provide the computational power needed to train and run complex AI models. NVIDIA's GPUs are also used in gaming, professional visualization, and other industries. The company's dominance in the GPU market has made it a key player in the AI revolution.
Axiom
Axiom is a data intelligence company that collects and analyzes publicly available data on individuals and organizations. It provides insights into people's demographics, interests, and behaviors, which can be used for marketing, sales, and other business purposes. Axiom's data sets are often used by companies to target their marketing campaigns and personalize customer experiences. The company's extensive data collection practices have raised concerns about privacy and the potential for misuse of personal information.
Q&A
What are some of the challenges Andrew faced when transitioning from finance to data analytics?
Andrew faced several challenges, including the lack of experience in data analytics, the need to work two full-time jobs to build his data resume, and the difficulty of finding a data analytics role that could fully replace his income from his finance position.
What are the key differences between teaching data analytics in academia and working in the industry?
Andrew notes that academia often focuses on the fundamentals of data analytics, while companies often require more advanced skills and knowledge of cutting-edge technologies. He also points out that many academic courses are outdated and don't reflect the latest advancements in the field.
What are the advantages of data lake houses over traditional data warehouses?
Data lake houses allow for analytics and AI to be performed directly on raw data, eliminating the need to move data to a separate data warehouse. This approach reduces costs, improves efficiency, and enables real-time AI and analytics.
What is Andrew's prediction for the future of AI?
Andrew believes that the future of AI will see a shift towards niche AI models that excel in specific tasks rather than large, general-purpose models. He also emphasizes the importance of data quality and the need for consistent definitions and accurate data to ensure that AI can be used effectively.
What is Andrew's opinion on the use of synthetic data for training AI models?
Andrew expresses reservations about the reliability of synthetic data, arguing that errors can propagate during training and exacerbate existing problems. He believes that there is no shortage of real-world data that can be used for training AI models.
What is Andrew's advice for people entering the field of AI or data analytics?
Andrew encourages people to start small and gradually build their skills, avoiding the temptation to jump into complex topics and become discouraged. He emphasizes that anyone can learn anything with dedication and effort.
What are some of the companies that Andrew believes will be the biggest winners in the AI landscape in the next three years?
Andrew highlights Google, Apple, and NVIDIA as potential winners. He believes that Google, despite recent missteps, has the potential to make a significant impact in the AI space, while Apple's integration of AI into its ecosystem could make it a major player. He also expresses a desire for a challenger to NVIDIA's dominance in the GPU market.
What is Andrew's perspective on the potential of open-source AI models to surpass proprietary models?
Andrew believes that open-source AI models have the potential to surpass proprietary models in the future, drawing parallels to the shift from SAS to Python and R in machine learning. He highlights the power of crowdsourcing and the potential for open-source models to achieve significant advancements.
What are some of the key takeaways from Andrew's discussion about the future of AI?
Andrew emphasizes the importance of data quality, the rise of niche AI models, the potential of open-source AI, and the need for companies to focus on building strong data foundations to ensure that AI can be used effectively.
Show Notes
In this episode, we dive into the fundamentals of AI modeling, discussing its core concepts and real-world applications. Join us as we explore how AI models are created, trained, and utilized across various industries.
My Podcast Course: https://podcaststudio.com/courses/Discount Code: AICHAT
Get on the AI Box Waitlist: https://AIBox.ai/
Join my AI Hustle Community: https://www.skool.com/aihustle/about